Yours personally
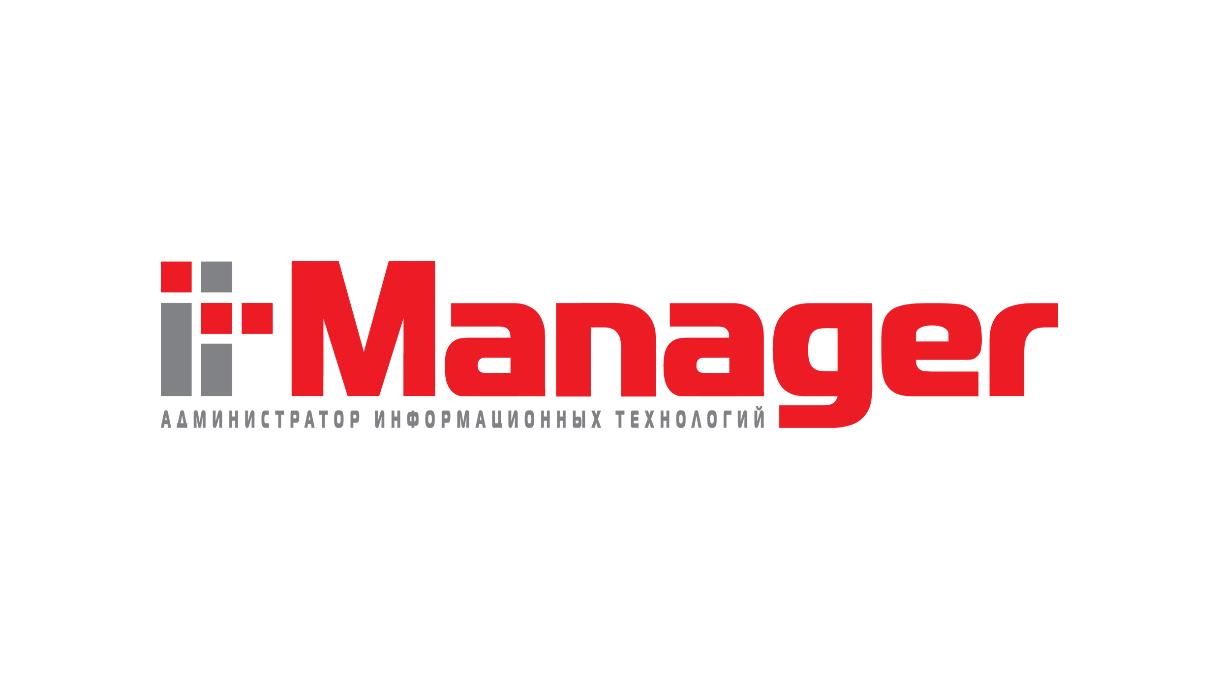
How machine learning can help forecast risks in motor insurance and customize insurance coverage.
As of the end of 2021, about 64 million passenger vehicles were crisscrossing the roads of Russia, almost twice as much as there used to be ten years ago. Cars are still the most popular transport in our country, but also the most dangerous: according to government statistics, last year alone, there were over 130 thousand road traffic accidents with casualties, and since compulsory third-party liability insurance for vehicle owners (MTPL) was enforced in 2003, the costs of covering damages are mainly borne by insurance companies.
We spoke about how machine learning can help forecast risks in motor insurance and customize insurance coverage with Cinimex’s key account manager Maxim Zharov, senior project manager Rodion Martynov, and Rosgosstrakh’s head of analysis and control department Olga Veresova.
Do AI and machine learning have a wide application in the insurance industry in Russia and abroad?
Maxim Zharov: No new disrupt technologies have emerged recently, it is just that machine learning is shifting from the hype stage into production. This is about systematization of the accumulated data, because every machine-learning model requires significant volumes of data, for at least three years. This data should be systemized and have enough depth. Basically, the main applications of ML for insurers haven’t changed: firstly, it is scoring and loss prevention, secondly, sales support and assessment of individual packages, and thirdly – pricing. All three are linked to policy customization. We are essentially studying what the customer wants and whether we can give them that.
Is it true that machine learning (ML) has a wider use in the western market, compared with Russia? And which parts of the insurance business can make the most of it?
Olga Veresova: Actually, both here and abroad, we have been using different types of modeling for quite a long time, for big insurers it has become a standard practice. Certainly, the insurance industry in Russia is not that mature due to its shorter history, but I believe that in terms of numbers of models, we are not that far behind. Domestic insurers use machine learning for the motor industry, because this tool can provide larger statistics, given a high number of cars and, hence, road accidents, and also because third-party liability insurance is mandatory. In the future, I think that ML will also find wide application in property and life insurance.
What are the barriers to a wider use of machine learning?
Maxim Zharov: The biggest challenge is to gather comprehensive and insightful data. If all you got is very scanty numbers, it is extremely difficult to build a model. Hypothesis can only be tested on large volumes of system data. Secondly, in a sense, ML is a study, and you can never guarantee a certain outcome. There will be an outcome, but not necessarily what the business hoped for. Thus, management can expect to receive specific information to back up development of new products or services, but even though it provides an extra tool of analysis, ML is not designed to make drastic changes in strategy. Besides, without proper formulation of a problem and a deep understanding of the specifics of business itself, and close coordination with the customer, all you can get is a set of basic characteristics, which will never lead you to a correct hypothesis, I insist.
Rodion Martynov: Let me add that machine learning has gone mainstream, it is actively implemented by manufacturing holding companies and retailers, above other. As for barriers, I would probably say high resource intensity. Neural networks require some very serious capacities. But now that over the last five years technologies have finally caught up with the methodology, ML has been rapidly growing in Russia.
How can ML be of use for businesses?
Olga Veresova: Such solutions can be of use to companies that are absolutely sure that machine learning fits their strategy as a means to build a model, which can serve your business for many years to come, even though the results won’t come overnight. Thus, our project is not a boxed solution, which can be simply purchased from the shelf. It is a product of a long-lasting joint effort with Cinimex.
The popularity of ML can sometimes cause inflated expectations, which are then followed by disappointment as to the potential benefits of this methodology for business. How can you avoid risks?
Olga Veresova: If you approach machine learning properly, it will help you grow your business. However, it is not a product that can guarantee a certain result in any scenario, such projects will also include a research part, and not all of your initial premises will turn out applicable. Plus, of course you need a highly professional team made of both business experts and ML engineers and analysts, who are experts in building correct models. In Rosgosstrakh, we have been doing it with good results for a long time now.
Can you tell us about the complex project between Cinimex and Rosgosstrakh? When did it start? What phases did it comprise? What was its specifics and who took part in it?
Olga Veresova: “Rosgosstrakh has been using models in the formation of MTPL and KASKO contracts, but there is always room for expansion. A year and a half ago we determined that we require a model that would ensure a more accurate risk assessment and major loss forecast using geography segmentation. This was how we partnered up with Cinimex. It is obvious to us that cars in Siberia and Moscow present a different level of risk, like when risks are not the same when you park your car in an industrial area or downtown. Previously, we only took into account the factor of region. That is why we reached out to Cinimex, so they would help us develop a model linking geolocation to each particular contract. Because every area has its specifics, which have to be factored in to the fullest extent possible. Apart from model development, a lot of technical work needed to be done: we purchased hardware, installed open-source software, and we hardly used any proprietary software, which is valuable in the current circumstances. As a result of the project, we obtained a service, a scoring module, which has been integrated into Rosgosstrakh’s pricing module since fall, and is currently used in quoting motor insurance contracts.
Maxim Zharov: The project kicked off in August 2020. There were a lot of tasks to address, so we moved forward step by step. The first thing we had to do is identify the impact of the area and its social and demographic profile on risks. There can be pedestrian crossings, traffic lights, video cameras, or objects that force drivers to speed down – all these factors allow to evaluate a specific area in terms of potential traffic accidents. We split the map into small fragments, and then geocoded the contracts against locations with registered loss-producing events, and then we started to juxtapose one against the other and search for additional risk factors that could be of use in the assessment of a particular insurance policy. This task was completed in the summer of last year.
Who took part in the project?
Rodion Martynov: On our end, we permanently had six-seven people working on the project, including project manager, administrator, team lead, data analysts and engineers.
Olga Veresova: Our company also assigned a numerous team, including both people from business divisions and IT – analysts, developers, specialists from data management department. They were engaged in the project in different periods, depending on their competencies. What is special about all ML-related projects is that they require significant input of time and high expertise. Standard methods, when everything goes as planned, do not work in this situation.
How does the geography segmentation model help to optimize risks? How precisely can you forecast the potential loss from a client?
Olga Veresova: This model is not self-sustained. We already count with a wide set of models that evaluate risk frequency and magnitude. Geography segmentation is a continuation of those models, it allows to significantly improve the forecast accuracy. I believe that in this project we were able to accomplish all tasks.
Rodion Martynov: Geography segmentation is one of the many factors that enable policy customization. Any insurance product is run through a certain pricing module that takes into account a number of factors, which can affect the price of a policy. Thus, the area factor, as we have already mentioned, helps to better the accuracy of risk assessment. Or, if a car previously had any suspicious history, for example, it was linked to people with confirmed abnormal activity, in this case our solution can help assess potential loss.
Can the system forecast sales growth potential?
Olga Veresova: Yes, it was the second goal of the project. With our system, we can figure out if we should open more sales points in this or that region.
Rodion Martynov: Let me explain, in our solution this is implemented through an interactive map, where you can zoom in down to a particular spot in the city where a sales office would work the best. We can zoom out or zoom in on the map and get recommendations on three different levels of viewing. The map highlights areas with good potential and those where a sales office would not be a good idea.
Do you already have any results?
Olga Veresova: Yes, the system has proven itself well. Even before putting any model into production, we test if it is worthwhile by retrotesting and AB-tests. What we do is analyze the client pool from the previous year, which generated income or losses, and apply the existing models enhanced with the new features to this pool of quotes, in order to find out if we would get an accurate risk assessment. Our models enhanced with a solution from Cinimex, give a more accurate assessment, which prevents us from signing potentially loss-making clients and enables us to set the best price for other clients.
Who will be responsible for tech support and updating the system?
Olga Veresova: We got two support lines: one is controlled by Rosgosstrakh, and the other one is currently held by Cinimex. Should a non-routine situation arise, we can always ask our colleagues for assistance. I believe that for one year following the deployment of a highly intelligent system this is an absolute must.
Rodion Martynov: We will continue monitoring the system. As for updates, the solution contains a certain procedure, so it self-updates all the marts and retrains models with control over all the metrics and deployment into production. The same goes for updating microservices, which ensure cross-communication between elements of the solution.
Can another insurance market player buy your system?
Rodion Martynov: As we said, this is not a box solution, this is custom software development for a specific customer, designed to address specific tasks. So all the artefacts of this project belong to our customer. Our system is impossible to copy.
Maxim Zharov: We are talking about a turnkey solution. Each company has their products and services, some of them sell better than other. We always start from exact numbers: sales numbers, scoring numbers. There is standard data applicable in any situation, but the devil is in the details. Every insurer wants to maximize their profits and minimize losses, but they can have different approaches as to how to make the best use of machine learning.
How will the system evolve? Will it get new functions?
Olga Veresova: Yes, we think it has potential for property insurance, for instance. We are connecting more and more analysts to the system. The pool of tasks to be addressed is big enough.
Rodion Martynov: Our project will be enhanced in terms of both tech support and new applications.