Every Ticket Will Find its Buyer: Demand Forecasting Systems for Stoloto
Cinimex developed and put into operation a system that improves the efficiency of lottery ticket distribution for Stoloto, the leading Russian distributor of state-run lotteries. Based on proprietary machine learning models, the system allows forecasting the number of tickets distributed through one of the largest retail channels – Russian Post offices.
We talked to Roman Romensky, Director of Sales at Stoloto, Kirill Dubovikov, Director of Technology at Cinimex Data Lab, and Maxim Zharov, Key Account Manager at Cinimex, about the role of machine learning technologies in modern businesses and the outlook for demand forecasting systems.
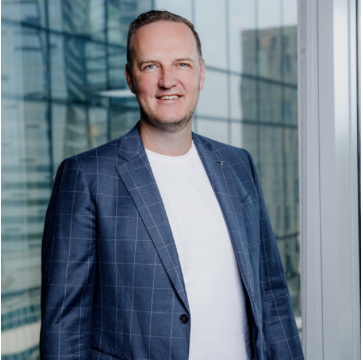
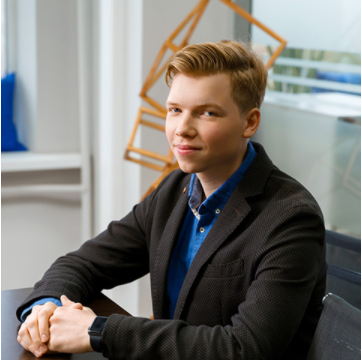
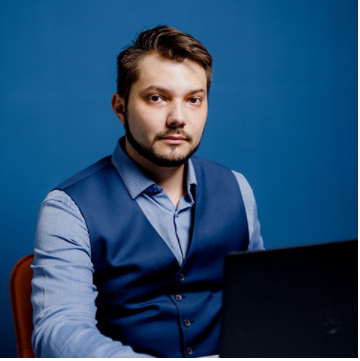
R&L: What kind of a system can increase the efficiency of lottery ticket distribution? How did this business process work before Cinimex’s solution was implemented?
R. Romensky: Today lotteries under the Stoloto brand are distributed in all 85 regions of Russia through more than 91,000 points of sale, including about 30,000 Russian Post offices. Calculating the optimal number of tickets to send to specific post offices was always a challenge for us. We were equally disappointed when there were not enough tickets, because our customers were not happy, and when many tickets were left unsold, because both for us and for the Russian Post this meant additional expenses on accounting, disposal, etc.
Together with Cinimex, we analyzed sales for the last three years, focusing on important events – holiday draws, the ones timed to Stoloto’s anniversary, and etc.
We discovered that almost every post office has their own patterns. A rural post office is a popular place, selling essential goods, and lottery ticket sales are very high there. Whereas an urban office mainly focused on parcel delivery tends to sell a limited number of tickets. Under the circumstances, it is nearly impossible to forecast demand manually. At this point we know for sure that rollover and holiday draws, especially New Year’s, sell the best. It is in these situations when the accuracy of the forecast is more important than ever: better to be overcautious than to leave customers without the tickets they desire, because otherwise, both the post office and us will be losing money.
This shows why machine-learning technologies are so valuable – they enable advanced analysis of retrospective data and its proper interpretation for the purposes of demand forecasting. After all, we start shipping tickets several months prior to the lottery date, so we need to have enough time to supply all post offices across all regions of Russia.
R&L: What data are used for the calculations?
R. Romensky: Just sales data for comparable periods.
R&L: How accurate is the forecasting now? Do you have a need for stockpiling?
R. Romensky: Of course, there is always a possibility of a force majeure, for example, if a big corporation decides to buy a massive amount of tickets for its employees from a post office. Anything can happen. For now, such extreme scenarios are not taken into account by the system, but in the future, they will also be factored in the calculations.
R&L: Why did you choose Cinimex to implement the solution?
R. Romensky: This one is easy – Cinimex's solution seemed to meet our expectations and needs at the possible best.
R&L: Is it too soon to evaluate the efficiency of the new solutions? Have any of the costs decreased after it was put into operation?
R. Romensky: It's still too soon, of course, but in the long run, we expect that the volume of tickets disposed of will decrease by at least 10%, and that tickets for next day's draw will be available for purchase at any office on the first half of Saturday.
R&L: How do you evaluate the usability and ease of integration of the proposed solution?
R. Romensky: By definition, such a complex solution, integrated into a huge network, cannot be simple. Nevertheless, the integration was completed within a reasonable time, and the product became available for use on the announced dates.
R&L: What were the stages of the project for the companies?
М. Zharov: The project kicked off a year ago. At the first stage, we collected data to confirm the hypothesis, and then we conducted a pilot project where we confirmed a certain relevance of the data for the distribution of specific tickets by points of sale, taking into account all the limitations (including logistics). It was determined how many tickets should be in a pack depending on the draw, seasonal and other factors. At the next stage we launched a full-fledged project, which since December was implemented through internal pilots in retail outlets. It had no influence on the decision-making yet – the information provided had advisory status. The final decision was made based on machine analytics and human expert evaluation. Once we made sure that the solution worked properly and had no negative impact on the existing business processes, we gave the go-ahead for its full-scale implementation.
R&L: Tell us what's under the hood of the solution.
К. Dubovikov: Under the hood of the solution, there is a machine learning system that calculates and evaluates hundreds of factors based on historical data. Sales history and marketing information provided by our customer are used as input data. The model training process is continuous, and the system allows us to train new models on demand in our UI.
The demand for tickets is broken down to every Russian Post office, taking into account the geographic information encoded into the training sample of our models. By specialized machine learning libraries we mean our time-series forecasting tool, which was developed as part of this project. We have implemented a flexible system that uses a number of forecasting techniques, which are not available in popular open source packages used for time series forecasting. In particular, we implemented specific solutions for model quality assessment in the situation of a large number of points of sale and hierarchical modeling tools tailored to customer needs. The system also allows combining different models depending on which one works better with each specific point of sale.
R&L: What does the interface of this solution look like? How user-friendly and intuitive is it? What is based on?
К. Dubovikov: Our user interface is divided into 2 parts: administrator interface, which allows to run forecast calculations and control their execution. It is a web application integrated with the customer's systems. And secondly, there is analytics interface, which is implemented as a BI Dashboard. This part of the system enables quick analysis of forecasts and sales for the entire company and individual points of sale.
When designing interfaces for data-driven systems, we strive to enable an ergonomic, user-friendly and valuable data visualization, drawing on what users of this information actually require.
R&L: What are the most promising applications for machine learning? What opportunities does this technology offer to customers?
М. Zharov: The most successful scenario from the point of view of further scaling is demand forecasting and logistics supply optimization – that is, determining the required volume of products for manufacturing, procurement, shipping to specific points, as well as setting a price on a product, seasonal factors, marketing campaigns, and etc. The solution Stoloto was based more or less on this type of scenario, which involves more than a hundred different parameters that significantly affect the decision-making process. Numerical data analysis represents the first layer of tasks, which can be addressed with the help of machine-learning technologies.
The second area of their application is security monitoring at a manufacturing facility and warehouse and POS inventory levels control employing computer vision and video analytics.
The third important application is related to wear and tear of conveyor equipment. Reconfiguration of such equipment is very labor-consuming.
R&L: Why does Cinimex rely on custom rather packaged solutions, does this approach provide any benefits to the customer?
М. Zharov: In terms of scaling, custom solutions most often win. When it comes to machine learning, out-of-the-box solutions need at least some customization, because each customer has a unique market position, unique data, unique business profile, and etc. If out-of-the-box solutions could satisfy all customers, there would be only one vendor on the market. But even with minor differences in tasks and requirements for the end result, any two companies will have completely different machine learning models requiring a different implementation approach.
Packaged solutions have an advantage – in the short term, they are cheap. In the long run, this advantage is offset by the need for external technical support, and besides, sooner or later, some customization will be needed anyway – and then you will have to ask for help from a vendor.
R&L: Can a custom solution be used by another company?
М. Zharov: We give the code to the customer as an intangible asset at the end of the contract along with documentation on the use of the system itself and all the algorithms. At this stage, the customer has to decide whether they want to work with the code by themselves, or want us to do the tech support.
We don’t use custom solutions on projects for another customer. We can build up our machine learning libraries and enhance the accuracy of forecast at a lower level of effort. In this case, the transfer of a readily available product is simply impossible, because each new project requires a new architecture built from scratch. Each deployment is, essentially, a large-scale consulting project that can never be a copy-paste of a ready-made solution.
R&L: What services for further maintenance of the solution does Cinimex offer the customer once the system is up and running?
М. Zharov: We offer two options. If the customer is competent enough to maintain the system, they take the technical support into their own hands. But, in general, companies prefer to outsource this task to us – it's easier and more advantageous economically in terms of the cost of additional training and other internal labor costs.
There are several scenarios of tech support – round-the-clock support or support during business hours; also, we have an option focused on addressing administrative issues, and another one stipulates involvement of data scientists or engineers should the customer need to make some minor adjustments. We guarantee that our model remains stable, despite any modifications and add-ons.
R&L: What other solutions does your company offer for retail and other industries?
М. Zharov: We have good experience in demand forecasting and optimization of logistics, as well as in delivering solutions for the financial industry (building buses, high load systems, infrastructure, microservices, scoring solutions, etc.). Besides, we are actively working with industrial companies for the implementation of computer vision technologies, among other. Our specialty is challenging projects, such as recognition of industrial products in warehouses to control their occupancy.
Occasionally, we are also faced with infrastructural tasks, such as data systematization and data engineering. Even if a customer have a need for data classification, they may not have a single dictionary within the company, or it’s distributed across multiple sources, which makes comparisons difficult.
R&L: What is the outlook for this technology?
М. Zharov: If we compare the current situation and the market three years ago, we see that companies have really stepped up their game in terms of data quality and enrichment. Previously, automation and machine learning were perceived as this R&D thing for the future, when the time is right. But today, companies already have accumulated a certain bulk of information that can be analyzed, or they are set on building a stock of data, which will then be used for different tasks.
At this point, machine learning is overcoming the hype around it to become a standard solution for any business that aims to thrive. As time goes by, this technology will be appreciated not only in large businesses, but also in the SME sector.
We were very lucky with the customer – this project was implemented in a very short time because we were able to build a large number of hypotheses from the available data, and whenever we found any issue, the feedback from the customer came immediately. This helped a lot when working on scripts, it felt like it wasn’t a machine-learning project, but just a regular IT project where everyone is well aware of the ultimate goal and outcomes.
R&L: How do you see the role of technology in modern business in general?
М. Zharov: What makes machine-learning technologies so valuable? First of all, they guarantee an unbiased assessment of the situation on the market and in the company. Most of the algorithms employ some sort of a scenario analysis – when the same situation is run through thousands of probabilities, and in the end, the choice is made in favor of the scenario that best matches the set of business rules and restrictions set by the company. The results can be factored into planning for more complex goals, such as differentiating from competitors, planning marketing campaigns, finding new points for growth, portfolio diversification, etc.
R&L: How do you evaluate the role of technology in Stoloto's business?
R. Romensky: Let me put it this way: ours is a very technological business, and those days when lotteries were associated with aunties selling tickets on the street are gone. A lottery is a 100% IT product, and ideally, we are going to build a complete ecosystem where you will be able to buy a ticket anywhere and claim your lottery winnings at any POS.